Neural mass models provide a physiologically grounded description of the average synaptic activity and firing rate of neural populations. First developed in the 1970s, these models are increasingly used for both local and whole-brain modeling in, e.g., epilepsy (Wendling et al., 2002; Wendling and Chauvel, 2008) or Alzheimer’s disease (Pons et al., 2010; Stefanovski et al., 2019), and for understanding and optimizing the effects of transcranial electrical stimulation (tES such as tDCS or tACS) (Molaee- Ardekani et al., 2013; Merlet et al., 2013; Kunze et al., 2016; Ruffini et al., 2018; Sanchez-Todo et al., 2018).
Neural Mass Models: An Overview
A neural mass model (NMM) is a mathematical model that describes the behavior of a large population of neurons in the brain as a single entity (Wilson and Cowan, 1972; Lopes da Silva et al., 1974, 1976; Jansen et al., 1993; Jansen and Rit, 1995; Wendling et al., 2002, Grimbert 2006).
These models simplify the complex dynamics of neural networks by considering the average behavior of a group of neurons rather than modeling the behavior of each individual neuron. In a neural mass model, the activity of the population of neurons is described by a set of differential equations that capture the interactions between different neural populations. These equations are derived based on experimental data and can be used to predict the behavior of the neural network in response to various stimuli.
NMMs are often used to study the dynamics of large-scale brain activity, such as the activity patterns observed in EEG and fMRI data–case in point in our group. They can also be used to study the effects of different drugs, diseases, and other perturbations, such as non-invasive brain stimulation (tDCS, tACS, TMS, etc. ) on the behavior of neural networks.
Overall, neural mass models provide a useful framework for studying the complex dynamics of neural networks and for understanding how changes at the level of individual neurons can affect the behavior of large populations of neurons.
Understanding Transcranial Electrical Current Stimulation (tES)
Transcranial Electrical Current Stimulation (tES, such as tDCS or tACS provided by our Starstim systems) is a non-invasive brain stimulation technique that involves the application of a weak electrical current to the scalp using electrodes. The electrical current is typically delivered using a battery-powered device that applies a low-intensity direct current. The electrical current in tDCS (“direct current” stimulation) can modulate the excitability of neurons in the brain, leading to changes in brain activity and function.
Depending on the direction and intensity of the current, tDCS can either increase or decrease neuronal activity in specific regions of the brain. tDCS is a relatively safe and well-tolerated technique that has been used in a variety of research and clinical applications. It has been studied as a potential treatment for a range of neurological and psychiatric disorders, including depression, chronic pain, and stroke. Some potential benefits of tDCS include improved cognitive function, enhanced motor learning, and reduced symptoms of depression and chronic pain.
However, further research is needed to fully understand the mechanisms underlying the effects of tDCS and to identify the optimal parameters for its use in different applications.
Modeling tDCS Effects using Neural Mass Models (NMMs)
Modeling the effects of tDCS using NMMs is interesting for several reasons:
Neural mass models provide a way to simulate the effects of tDCS on large-scale brain activity. These models allow researchers to predict how tDCS might affect the activity of neural networks and to understand the underlying mechanisms of tDCS-induced changes in brain function.
NMMs can help to optimize the parameters of tDCS, such as the location, intensity, and duration of stimulation. By simulating the effects of tDCS using these models, researchers can identify the optimal stimulation parameters for specific applications and optimize the efficiency of tDCS protocols.
Neural mass models can be used to study the effects of tDCS on brain disorders and diseases. By simulating the effects of tDCS on neural networks affected by these disorders, researchers can identify potential targets for tDCS treatment and improve our understanding of the underlying pathophysiology of these disorders.
Overall, the use of neural mass models in tDCS research provides a powerful tool for understanding the effects of tDCS on large-scale brain activity, optimizing stimulation parameters, and identifying potential targets for the treatment of brain disorders.
Our team recently published a couple of papers on an extended version of NMMs called the “laminar” formalism, and that includes physical elements to better connect with measurements such as EEG, SEEG (Lopez-Sola et al., 2022), or multiprobe measurements (Sanchez-Todo et al., 2023). Stay tuned, these will be the subject of future posts!
Here we address a different aspect: NMMs are only partly derived from first principles. They represent effective theories – semi-empirical models derived from observations. This means it is not always clear how to set some of their parameters (e.g., coupling with an electric field, see Figure 1) or what the limits of their applicability are.
Exact Mean-Field Theory for Quadratic Integrate-and-Fire (QIF) Neurons
In 2015, Montbrió et al. (MPR, 2015) derived an exact mean-field theory for networks of quadratic integrate-and-fire (QIF) neurons, thereby connecting microscale neural mechanisms with mesoscopic brain activity. Within this framework, the response of a neural population is described by a low-dimensional system representing the dynamics of the firing rate and mean membrane potential.
Therefore, the MPR equations can be seen to replace the usual static transfer sigmoid function with two differential equations grounded on the biophysics of the single neurons. Since then, the theory has been applied to cover increasingly complex formulations.
NMM1 vs NMM2
A new research paper (Clusella et al. 2022) from our team, led by the Neurotwin UPF group, has recently shed light on the collective dynamics of neuronal populations in the brain. The study analyzes and compares two neural mass models (NMMs) that are commonly used to study these dynamics. The traditional model, known as NMM1, is a heuristic approach along the lines of the original NMM work that assumes the output firing rate of a neural population can be described by a static nonlinear transfer function.
In contrast, the new exact mean-field theory for quadratic integrate-and-fire (QIF) neurons, known as NMM2, proposes that the mean firing rate follows two coupled nonlinear differential equations.
Our study investigates the applicability of these models in the presence of second-order synaptic dynamics, which are essential for understanding how neurons communicate with one another. We show that NMM1 fails to accurately capture the complex dynamics of neuronal populations compared to NMM2, particularly in the presence of fast inhibitory or excitatory synapses (where fast is relative to the membrane time constant).
The exact model, NMM2, displays rich dynamics (see Figure 2) and reveals previously unknown phenomena that NMM1 was not able to capture, such as self-sustained oscillations of an inhibitory interneuron QIF network and resonant oscillatory activity induced by stimulation of a pyramidal cell population.
Conclusion
These results are significant because they emphasize the importance of using more accurate models to study the collective dynamics of neuronal populations. These models could provide new insights into brain function and help design more effective brain stimulation techniques. In particular, the study highlights the potential of resonant oscillatory activity induced by brain stimulation to enhance the response of densely connected networks to weak uniform inputs.
This finding could help optimize brain stimulation techniques and improve their effectiveness in treating various neurological disorders such as epilepsy (the subject matter of the galvani-lab.eu project) or Alzheimer’s disease (neurotwin.eu).
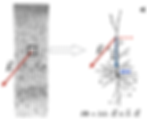
Figure 1: One of the important challenges in modeling tDCS is to understand the network effects of weak electric fields that have a small impact at the cell level but lead to mesoscale changes due to network effects.

Figure 2: NM2 (E-I model) can generate beautiful dynamics (v. Ruffini2022 for more).