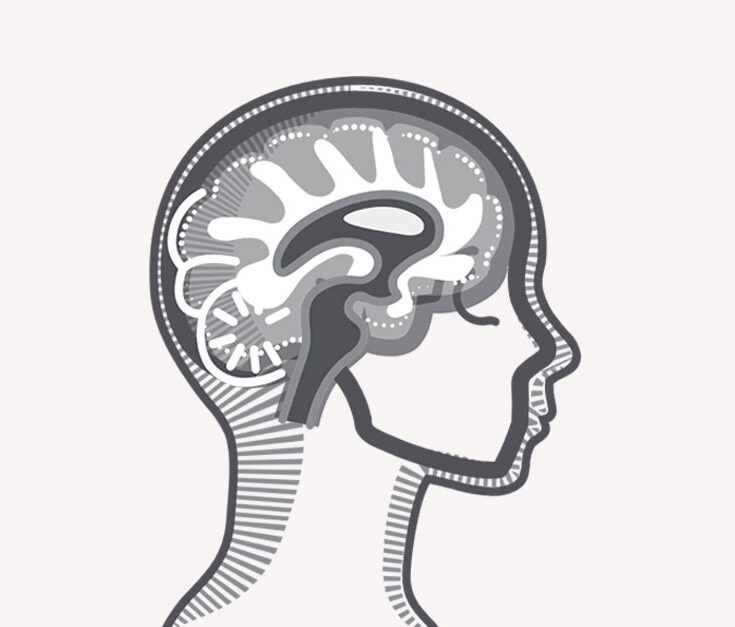
Neuroelectrics is revolutionizing brain therapy through personalized neuromodulation therapies. A cornerstone of this innovation is the development of Neurotwins, which are personalized digital replicas of the brain.
In this first part of our blog series, we will delve into the intricate process of creating neurotwins, from data acquisition to the fine-tuning of personalized montages. Essentially, we will explore the journey from patient data to a set of electrodes placed in specific positions and currents with given waveforms.
Our discussion will focus on two types of models:
Biophysical head models (tES 1.0): These models, already available in the market, simulate the flow of electrical currents through a detailed representation of the head, accounting for various types of tissue.
Neurotwins (tES 2.0), Building upon the foundation of biophysical models, tES 2.0 introduces more advanced simulations, focusing on how neurons, in addition to tissues, react to the electric fields generated. We will explore this further in our next post.
Tip: For those interested in the fundamentals of tES, you can find more information here, along with a short introduction to the models we use here.
Biophysical head models (tES 1.0)
Biophysical head models (tES 1.0) serve as the backbone of our personalized neuromodulation therapies, constructed exclusively from biophysical data, primarily derived from magnetic resonance imaging (MRI). These models play a pivotal role in simulating electric fields for precise electrode montages tailored to each individual anatomy.
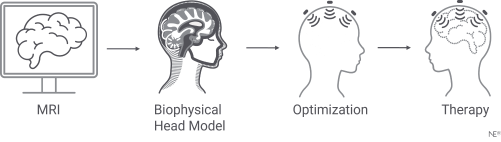
Tip: You can complement this information with the Modeling Services page
Key Concept: What is MRI? MRI, short for Magnetic Resonance Imaging, is a non-invasive method for producing 3D detailed anatomical images. It’s commonly used in medical settings for detecting diseases, diagnosing conditions, and monitoring treatment progress. This technology uses powerful magnets to align protons within the body with a magnetic field. By applying radiofrequency pulses, the protons are stimulated and subsequently emit energy as they return to their original alignment. This emitted energy varies depending on the tissue type, allowing physicians to differentiate between different tissues and diagnose accordingly [1]. |
This process is akin to capturing a satellite image of a landscape – enabling us to identify key landmarks and gain a general understanding of the terrain's characteristics.
Key Concept: What is image segmentation? The process of identifying and labeling each tissue or group of tissues is called segmentation. In fact, this concept is general across image processing and does not need to refer only to medical images and biological tissues. Image segmentation can be done manually by having some operator mark each tissue one slice at a time or be (partially) automated. In Neuroelectrics, we have developed our own algorithms to segment it automatically, with little manual intervention, with the help of deep neural networks [2]. |
Following the previous metaphor, segmentation would be equivalent to taking a satellite image highlighting the different zones – the countryside, the water bodies, and the bustling city streets.
Picture this!
Let’s say we’re checking out a map of the area around Barcelona. With image segmentation, we can spot the various landscapes – the vibrant city vibes, the tranquil countryside, and the sparkling blue waters along the coast.
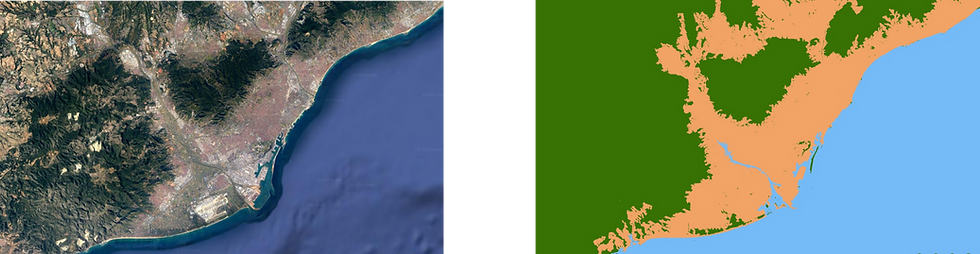
Fig 1: Example of a satellite image of Barcelona and its surrounding areas (left, Google Earth) and segmentation into urban areas (orange), bodies of water (blue), and countryside (green).
The key point for personalization is that segmenting an MRI enables us to produce a 3D model of the brain with distinct tissues.
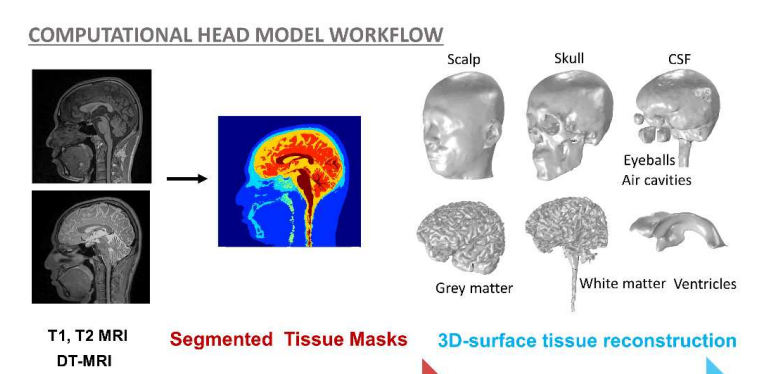
Fig 2: Example of an MRI segmentation and 3D-surface tissue reconstruction. Adapted from [3].
Picture this!
Why are the different tissues important to identify to build neurotwins? Imagine you want to send a message to Atlantis, the city under the sea. To do so, you place a powerful speaker from a boat and orient it towards the bottom of the ocean. As it emits sound, the message dissipates more rapidly in the water than in the air. Only a very small signal would reach the city!
Similarly, just as sound dissipates differently in water compared to air, electric currents propagate differently through various brain tissues. When building a personalized stimulation protocol, this is one of the first things we need to understand.
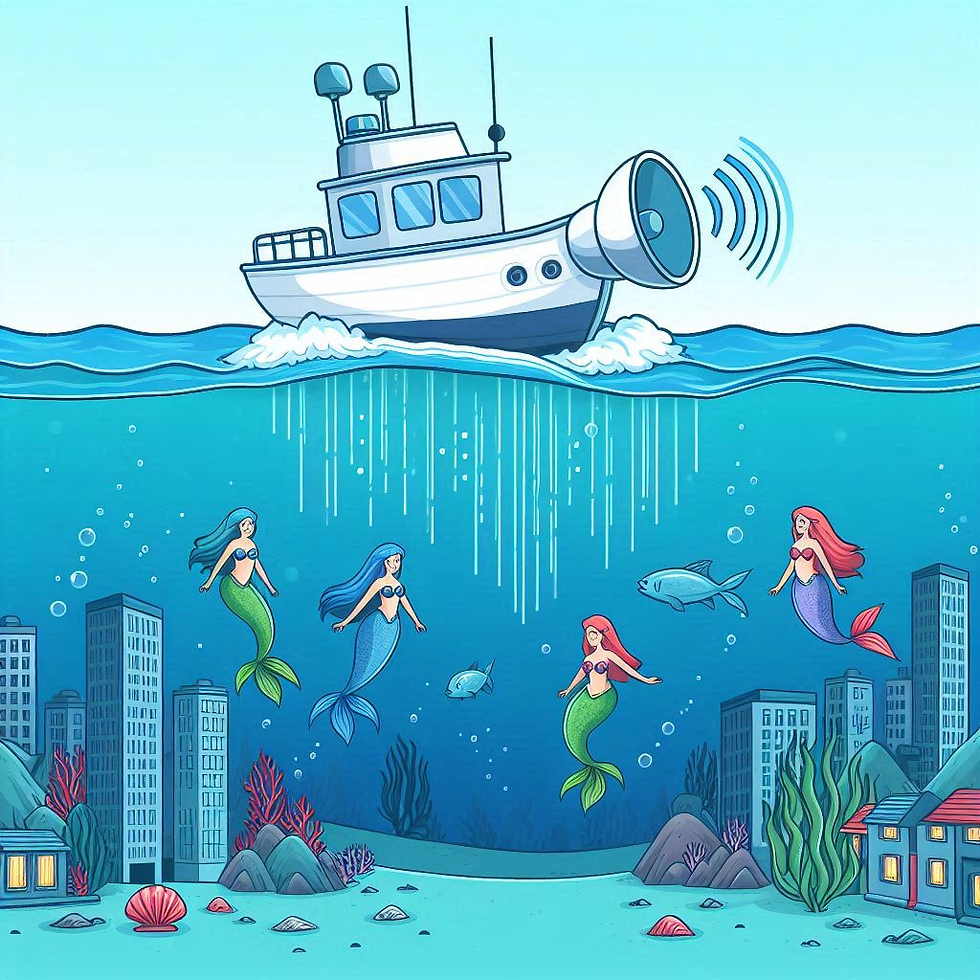
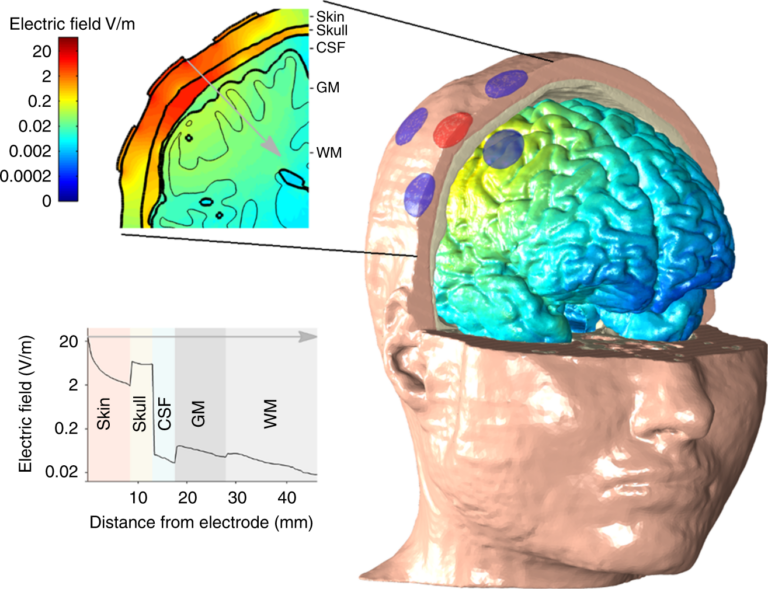
Fig 3: Left: Artistic interpretation of a boat sending a message to Atlantis. Created using Dall·E 3. Right: Electric field propagation on the different tissues. Taken from [4].
To be more precise, sound travels around four times faster in water than in air but it is more attenuated by the former, since it has a higher viscosity. On top of it, higher frequencies are more attenuated than lower frequencies. You can read more about it on Wikipedia’s page for Stoke’s law of sound attenuation!
In our models, we distinguish up to 7 different tissues: the skin, skull, cerebrospinal fluid (CSF), gray matter (GM), white matter (WM), the eyes, and the ventricles. Each of them has a different electrical conductivity, that is, a different ability to conduct electric currents. For instance, bone (the skull) poses more resistance to the electric current than the gray or even the white matter.
Once the image is segmented, we construct a mesh, i.e. a set of triangles (i.e. nodes and faces) that closely approximate the 3D volumes. Each node then has a label assigned to it regarding the tissue it belongs to. A typical model has hundreds of thousands of nodes, which constitute the very detailed mesh needed to capture the brain’s sulci and gyri (the folds and grooves).
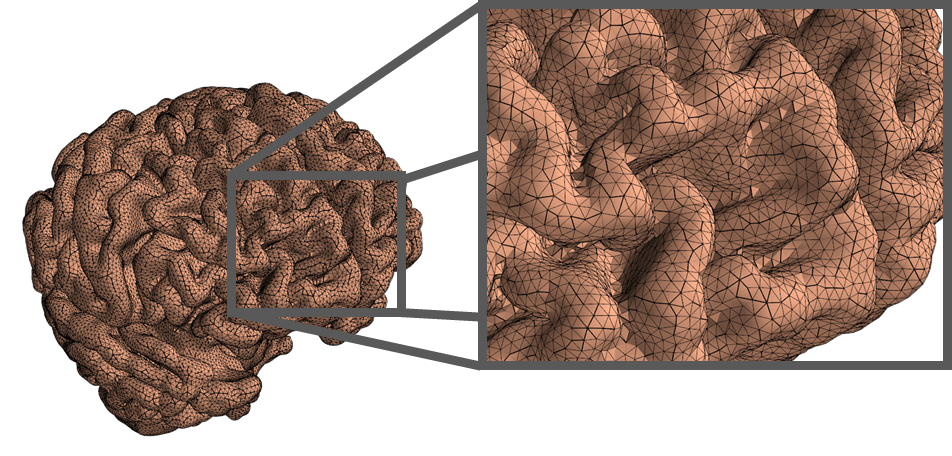
Fig 4: Example of a gray matter mesh and zoom to appreciate the triangular mesh.
All this – the labeled mesh and the conductivities – constitutes the biophysical head model. With it, we can use finite element methods (FEM) to simulate the propagation of the electric currents and, thus, the electric field induced in each node given a pair of electrodes.
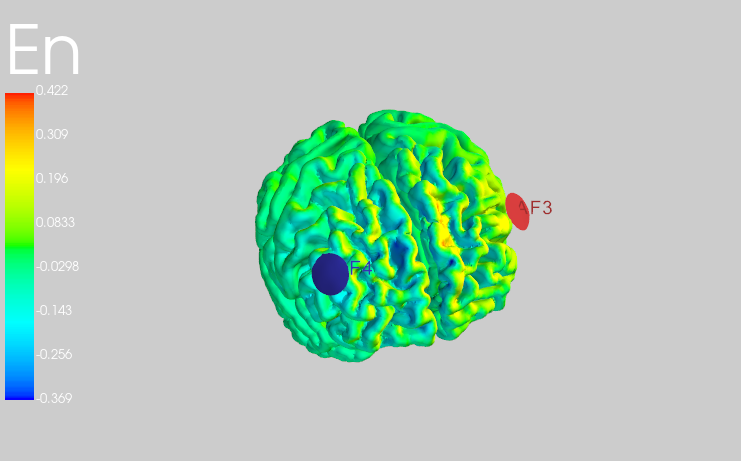
Fig 5. Electric field simulation, specifically the component normal to the cortical surface, for a montage with currents of 2 mA in the AF3 (anodal) and AF4 (cathodal) electrodes.
Key Concepts: What are finite element models (FEM)? Finite element models (FEM) provide a powerful computational tool for solving complex problems like electric field propagation. By breaking down the head model into smaller parts or triangles, FEM approximates how electric currents and fields propagate in the brain model using Maxwell’s equation. This technique, not limited to electric fields, finds applications in various fields, including structural analysis, heat transfer, and aerodynamics. |
Optimizing a montage using a biophysical head model only: StimWeaver 1.0
For this kind of tES personalization (1.0), montage optimization is typically based on a target map defined by the expert (e.g., a clinician): an area or areas of the brain to be stimulated, inhibited, or unaffected.
Tip: Check the wiki page for more information
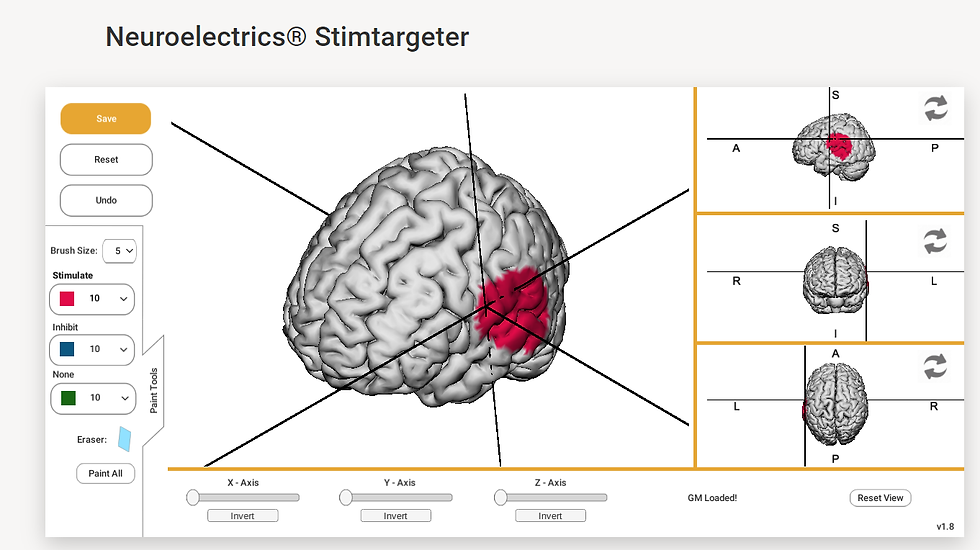
Fig 6: Neuroelectrics' Stimtargeter
StimWeaver (1.0) is the optimization service created by Neuroelectrics designed to create stimulation montages (electrode positions as well as currents) specifically adapted to user-defined targets. Finding the best electrodes’ position and currents constitutes a combinatorial optimization problem.
It may be counterintuitive to some, but the challenge lies not in determining the current values but in finding the optimal positions for the electrodes. Indeed, for fixed electrodes’ position, it is straightforward to find the best currents. The only way to ensure a global maximum – that is, the absolute best solution – for the electrodes’ position would be to try all possible combinations. Unfortunately, it would take a big computational toll! For instance, for a Starstim 32 (32 possible electrode positions) and a restriction of up to 6 electrodes being used, there are almost 1 million combinations! However, we can exploit some clever search strategies to obtain a good solution in a reasonable amount of time. For instance, genetic algorithms which take inspiration from natural selection, provide a great trade-off between performance and are the ones incorporated in StimWeaver [5].
For 32 possible positions, we have binomial(32, 6) possible combinations when choosing only 6, which roughly approximates to 900,000.
Learn more: Optimization problems and genetic algorithms Let’s deep dive into the two aspects of our combinatorial optimization problem: 1. finding the best electrodes’ position, which is the combinatorial part, 2. finding the best currents given these electrodes, which is a constrained linear optimization problem. As we outlined in the main text, finding the currents is easier than the positions. This is due to the nature of the problem: – Constrained, since we need to impose conditions on the currents, such as a maximum allowed current per electrode and a maximum total current. – Linear, which means that there is a linear relationship between the currents and the value we want to optimize – i.e., the electric field on the target. – Convex, which means that there is only one local maximum These types of problems can be solved using constrained least squares, which provide a global maximum – “an absolute best” solution – with a reasonable amount of effort. As for the electrodes’ position, since it is a combinatorial problem, the general strategy is to try different combinations for a number of iterations and keep the best result. Here, different algorithms can help to find a good enough combination with fewer iterations by choosing what combination to try next in a clever way. |
Learn more: Stimweaver [5, 6] Stimweaver is an optimization method designed for enhancing multichannel transcranial electrical stimulation (tES). Its goal is to improve cortical excitability by optimizing the placement and intensity of electrodes. To achieve this, it focuses on the component of the electric field perpendicular to the cortical surface, which according to the lambda-E model [7] – inferred from prior in-vivo and in-vitro work – is one of the key interaction mechanisms. |
Conclusion
In this first part of our series, we’ve explored the creation of Neurotwins through biophysical head models. Using advanced imaging techniques like MRI and sophisticated image segmentation algorithms, we develop detailed 3D brain models that simulate electrical currents with high precision, tailoring electrode montages to each individual’s anatomy for optimized personalized neuromodulation therapy.
The biophysical head models (tES 1.0) represent a significant advancement, allowing precise targeting of specific brain areas with optimized electrode placements and currents, crucial for effective neurological treatments. As we move forward, the integration of more sophisticated simulations in Neurotwins (tES 2.0) will further enhance our understanding and influence of brain activity. Stay tuned for the next part, where we explore these advanced simulations and the introduction of Neurotwins (tES 2.0), providing a deeper understanding of neuronal reactions to electric fields.
References:
[1] Magnetic Resonance Imaging (MRI) National Institute of Biomedical Imaging and Bioengineering. https://www.nibib.nih.gov/science-education/science-topics/magnetic-resonance-imaging-mri
[2] Salvador et al., Deep neural networks for MRI segmentation, In prep.
[3] Miranda, P. C., Callejón-Leblic, M. A., Salvador, R., & Ruffini, G. (2018). Realistic modeling of transcranial current stimulation: The electric field in the brain. Current Opinion in Biomedical Engineering, 8, 20-27.
[4] Asamoah, B., Khatoun, A., & Mc Laughlin, M. (2019). tACS motor system effects can be caused by transcutaneous stimulation of peripheral nerves. Nature communications, 10(1), 266.
[5] Ruffini, G., Fox, M. D., Ripolles, O., Miranda, P. C., & Pascual-Leone, A. (2014). Optimization of multifocal transcranial current stimulation for weighted cortical pattern targeting from realistic modeling of electric fields. Neuroimage, 89, 216-225.
[6] Ruffini, G., Wendling, F., Sanchez-Todo, R., & Santarnecchi, E. (2018). Targeting brain networks with multichannel transcranial current stimulation (tCS). Current Opinion in Biomedical Engineering, 8, 70-77.
[7] Ruffini, G., Wendling, F., Merlet, I., Molaee-Ardekani, B., Mekonnen, A., Salvador, R., … & Miranda, P. C. (2012). Transcranial current brain stimulation (tCS): models and technologies. IEEE Transactions on Neural Systems and Rehabilitation Engineering, 21(3), 333-345.
Images:
All images are either referenced, generated in-house or, in the case of the illustrative drawings, generated by Dall·E 3.